Katana Labs IP
Background
Developed in-house at ING, Katana was a project conceived to assist portfolio managers and traders in their day-to-day decision-making processes.
​
The platform identified unconventional relative value opportunities in the bond market, specifically catering to institutional traders and portfolio managers.
Proving effective during its preliminary stages, this pre-trade analytics tool evolved from a prototype to a market-ready product in just over a year. Recognizing its potential, ING decided to launch Katana as an independent business entity in 2019.
Katana's Method For Relative Value
When dislocations emerge in bond pairs with historical price/spread trends, and high similarity attributes, Katana advises trading the cheaper bond to capitalize on the market correction.
​
Katana uses machine learning models to power the three main processes: identifying relevant pairs by pricing and attribute patterns, assigning a Z-score for the spread difference of the pair, and predicting the compression.
To determine if bonds may be over or undervalued relative to their counterparts, the algorithm utilizes end-of-day credit spread data (Z-spread) as the pricing metric.
The platform utilizes a combination of steps to assess whether or not a given bond pair has been statistically related in the past. Similarity attributes, such as maturity, duration, seniority, currency, country of risk, credit rating, and sector help identify bonds and provide a single similarity score.
​
In production, Katana processes nearly 5 million distinct bond pairs daily, depending on the size of the universe.
​
The model outputs a Z-score for the current spread difference of the pair, compared to historical data. The Z-score measures the number of standard deviations the pair's spread difference is from the past year's historical mean. A higher Z-score indicates a more significant deviation from the norm.
​
Katana's deep neural network (DNN) predicts the likelihood of spread compression, estimating the probability of the pair's spread difference reverting to its mean. Compression on dislocations has been shown to be approximately 90% accurate over a measured period of 30 days.
​
In March 2021, the front-end technology was integrated into the Bloomberg Terminal's application portal (APPS<GO>), enabling potential seamless integration for over 330,000 Bloomberg users.
Opportunities Generated on January 5, 2022
Looker Studio Prototype
Legend for Bond Market
IGUS - USD Investment Grade
USHY - USD High Yield
IGEUR - EUR Investment Grade
SSA - EUR Sovereign, Supranational, and Agency
EMD - USD Emerging Markets
EURHY - EUR High Yield
EGR - EUR Government
Opportunities Generated on January 28, 2022
Looker Studio Prototype
Use the drop-down menus to narrow the list down to a specific Market, Sector Or Maturity Year
Legend for Bond Market
IGUS - USD Investment Grade
USHY - USD High Yield
IGEUR - EUR Investment Grade
SSA - EUR Sovereign, Supranational, and Agency
EMD - USD Emerging Markets
EURHY - EUR High Yield
EGR - EUR Government
Bloomberg Terminal Users Can Click On Green Function Buttons
Examples of Application Features and Opportunities
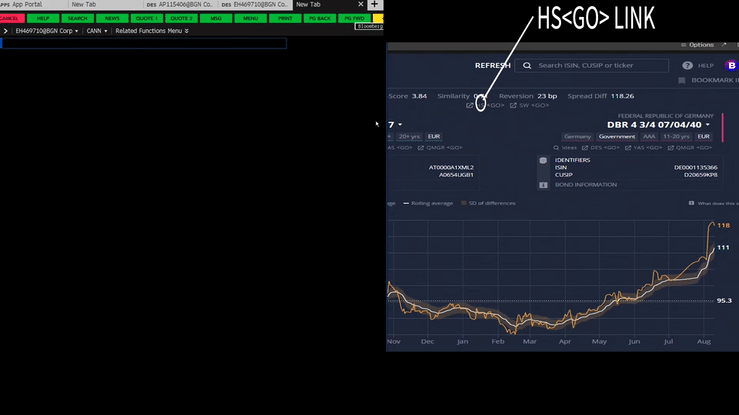
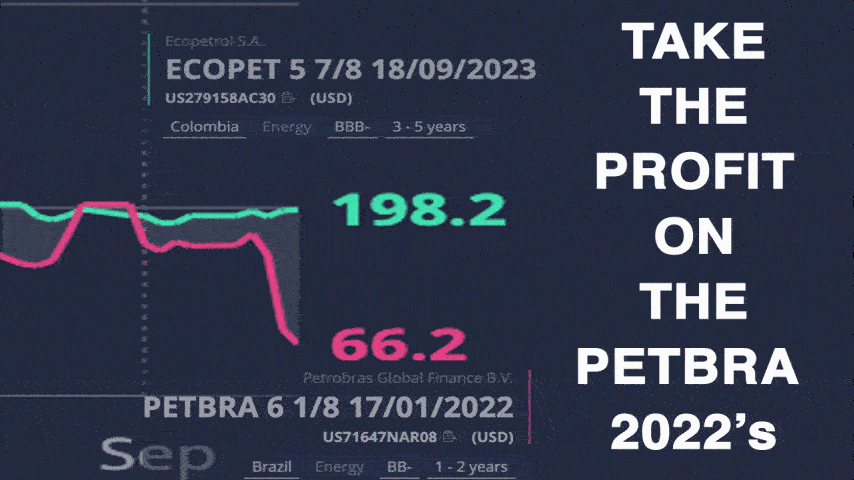

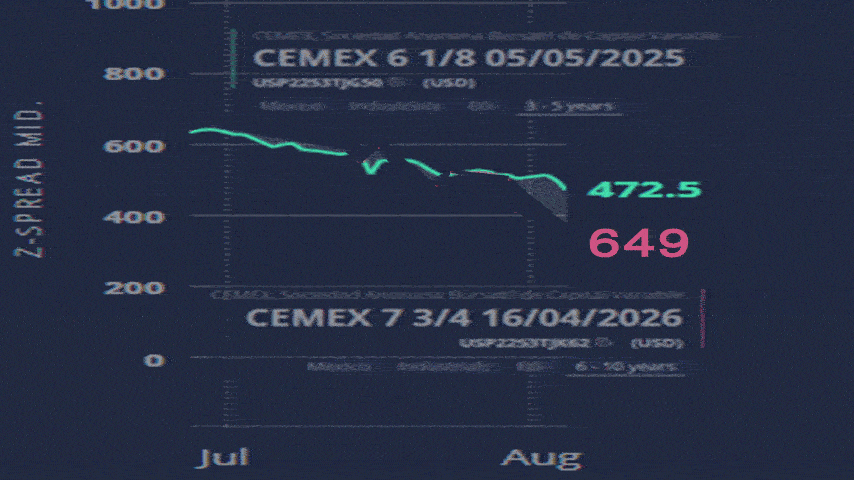
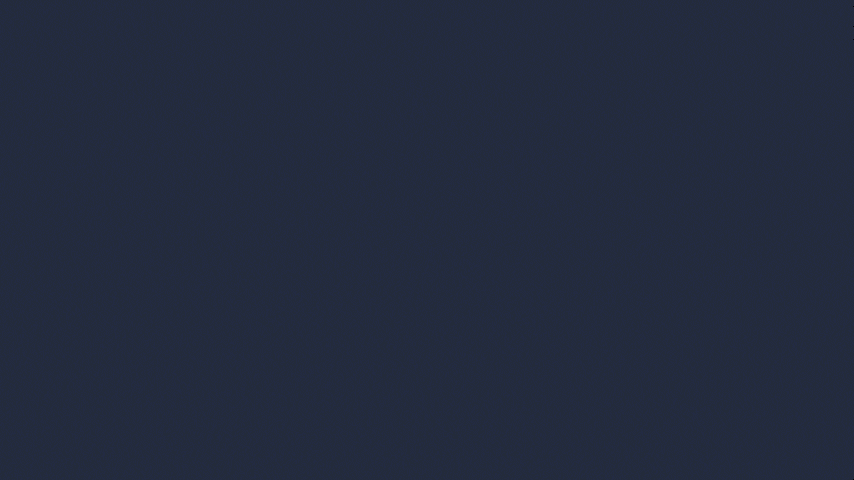
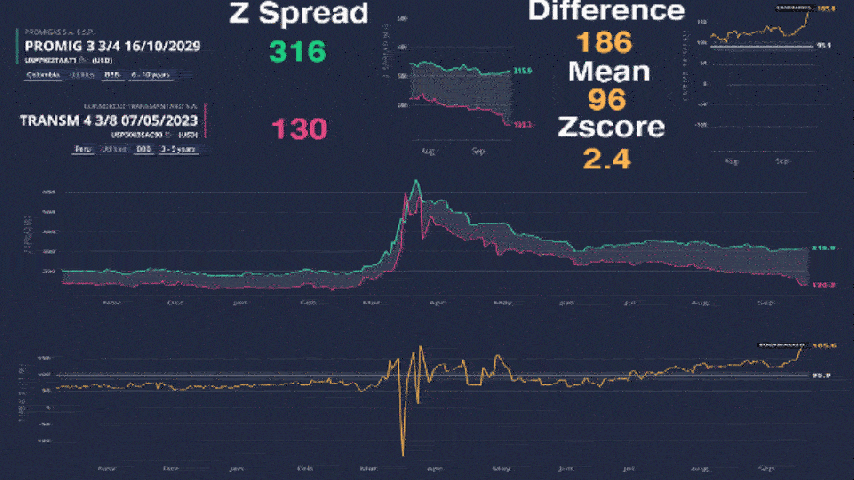
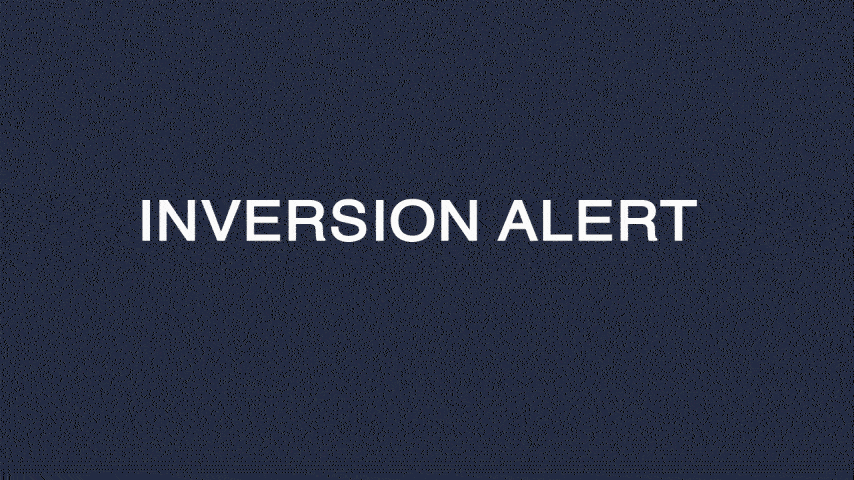
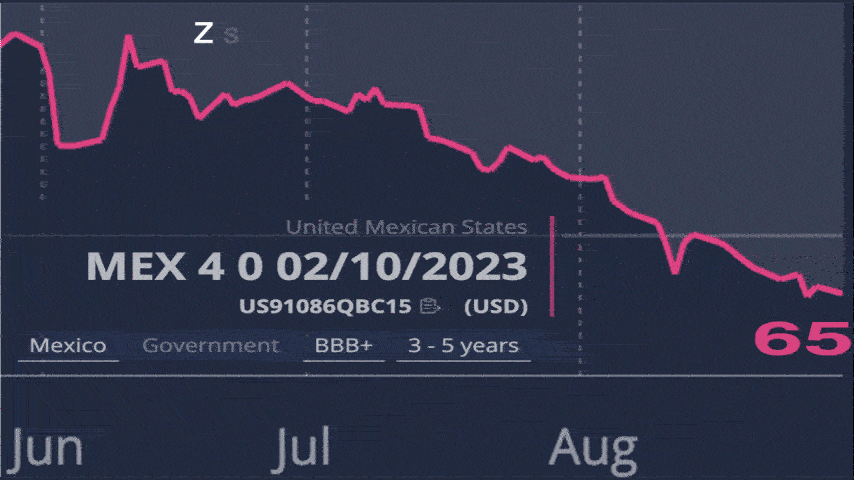
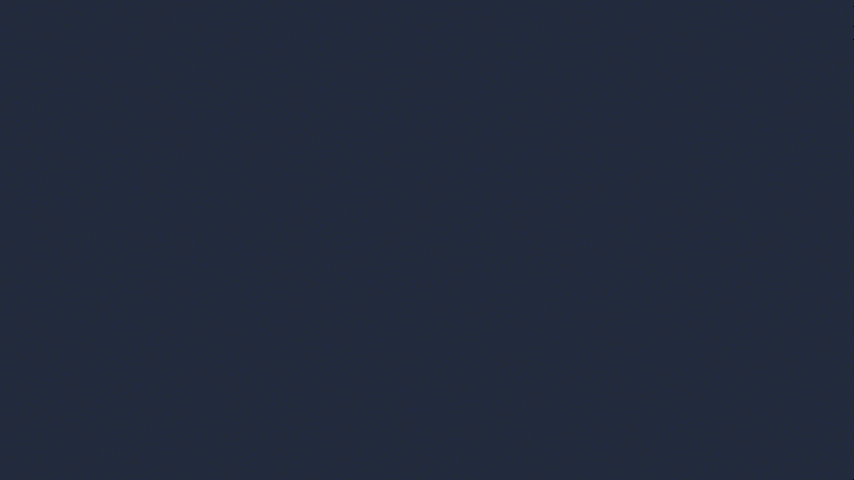

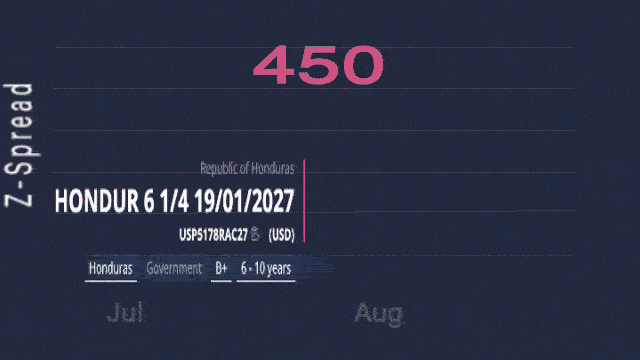
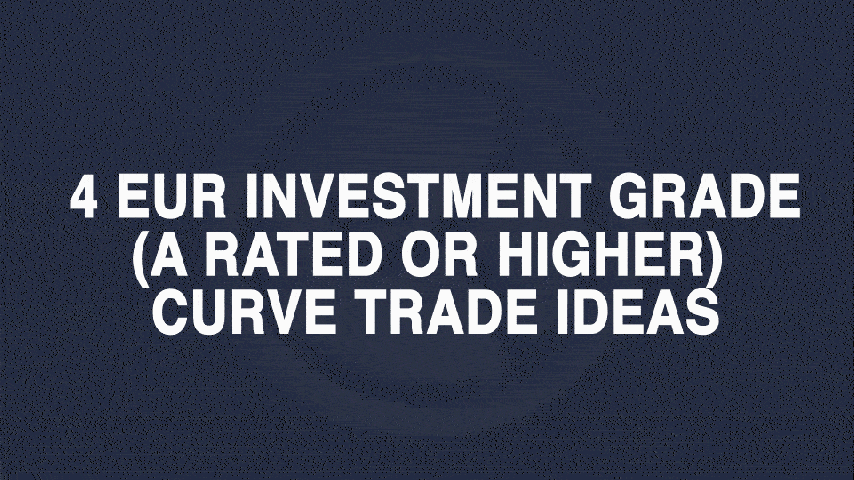
A Sample of a Daily Dislocation Email
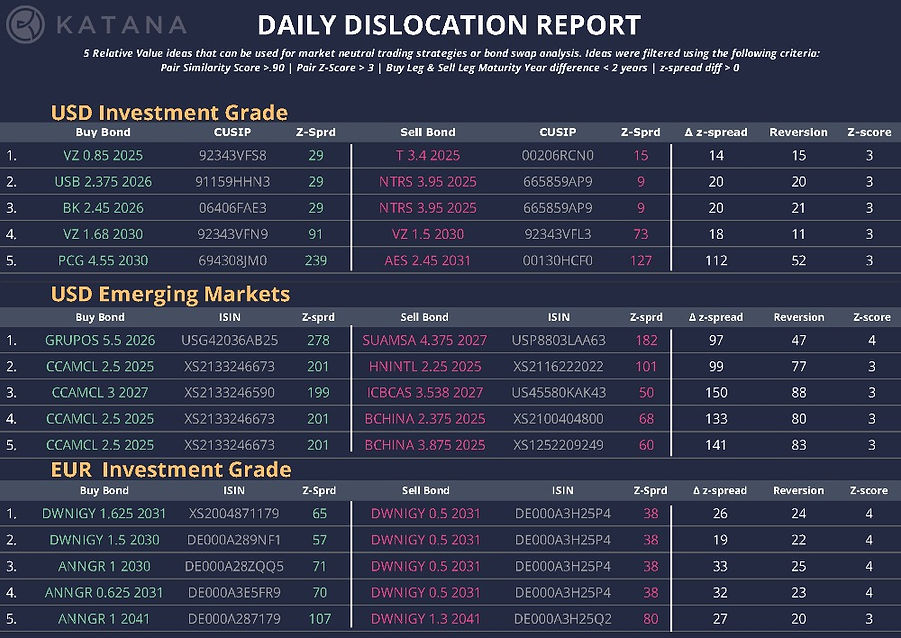